Online Trust: An International Study Of Subjects’ Willingness to Shop at Online Merchants, Including The Effects of Promises and of Third Party Guarantees
Online Shopping E-Commerce Android App Project
Online Trust: An International Study Of Subjects’ Willingness to Shop at Online Merchants, Including The Effects of Promises and of Third Party Guarantees
Significant differences exist among consumers’ online shopping behavior in different international markets. This paper compares consumer behavior in four dif-ferent markets: (1) The US, the largest and most ma-ture market, (2) Germany, a similarly advanced west-ern market (3) China, the fastest growing online mar-ket, but one where consumers are plagued by incidents of counterfeits, forgeries, and spoiled or defective items, and (4) Singapore, an advanced market, cultur-ally similar in some ways to China, but with a strong legal system. We performed laboratory experiments simultaneously in all four countries. We used three experimental treatments:(1) No assurances of product quality or authenticity, (2) promises of quality and authenticity, and (3) promises backed up by third party assurances. We examined subjects’ responses for all three treat-ments, and for vendors’ with different degrees of riski-ness. We confirmed that significant differences exist in consumer behavior, but these differences were not always what we expected. Chinese consumers do appear to have trust in their best online vendors. US consumers appear to treat online shopping very simi-larly to the way they treat shopping in physical venues.
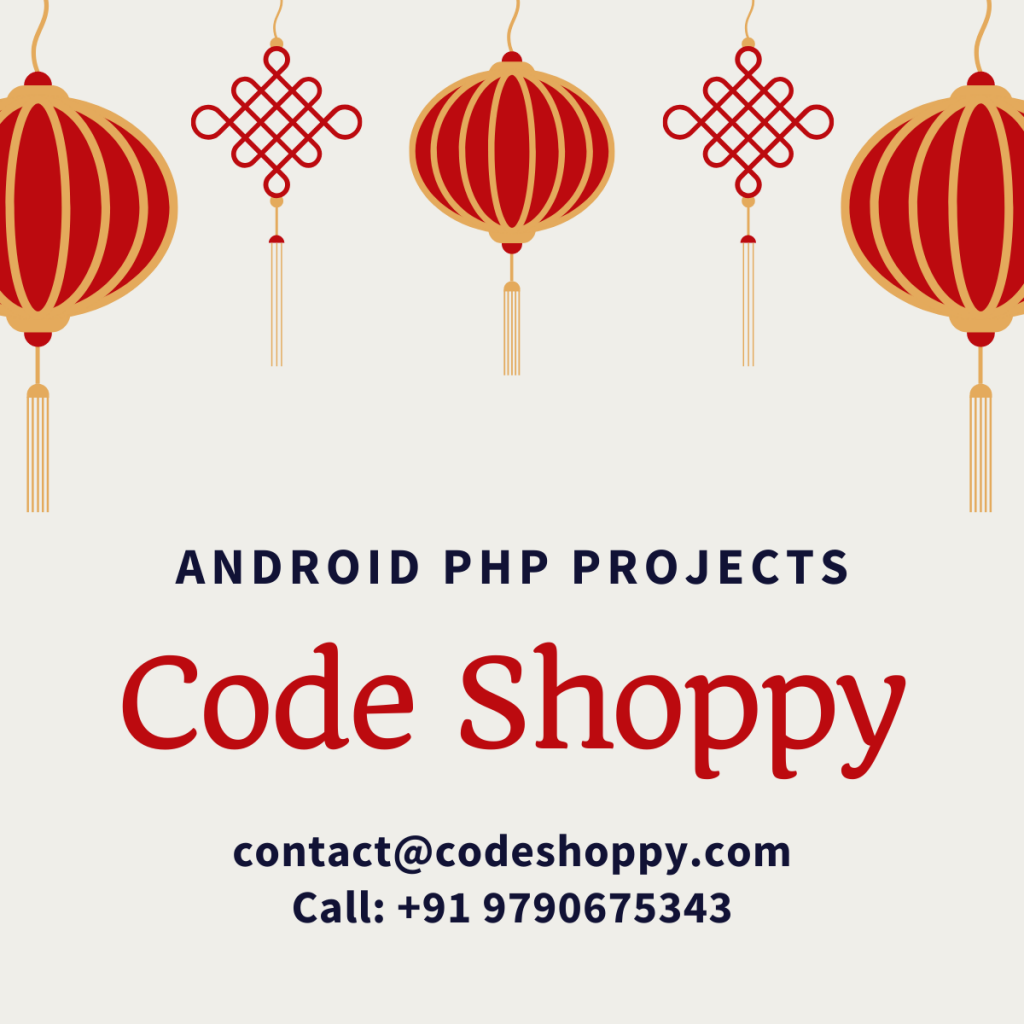
The experiment is firmly grounded both in theory and in practice. It draws on the literature on infor-mation asymmetry, and on signaling mechanisms and reputational capital as mechanisms for dealing with information asymmetry. It draws also on experimental studies of indicators that consumers use to assess the relative risk of different online sellers. Our experi-mental design has been described in detail in prior publications [5]. We review essential details here. Subjects were asked to assess their WTP for 10 or 11 different products from a set of the same merchants, under two treatments out of the three shown in Table 1. Subjects were shown only the two treatments rele-vant to their participation in the experiment. Subjects could choose not to express a WTP for any product they did not have an interest in purchasing. Those subjects who were interested in a product and who expressed a willingness to buy it online from at least some vendors, could choose which vendors they would purchase from and under which treatment. That is, we allowed subjects to select some vendors but not all vendors, based on their perception of risk from indi-vidual vendors and their own risk preferences. This is consistent with subsequent work by Gu et al., [33], on elimination by aspects, showing that Chinese consum-ers would first accept or reject a merchant based on perceived riskiness; they would then express a WTP only for those remaining merchants deemed acceptable to them. Subjects were shown a photograph of the product and a description of the product as would be found on a trusted vendors’ site. The subjects were given two reference prices, the manufacturer’s suggested retail price and an average online selling price from a highly respected seller. Subjects were then asked to provide what they would be willing to pay for the product, from the set of sellers, under two of three treatments: 1.(MIN) Subjects were asked the amount that they would be willing to pay for the product from each of the described vendors, with no specific assur-ances from the vendor concerning product quality or authenticity. 2.(MID) Subjects were asked the amount that they would be willing to pay for the product from each of the described sellers, in the presence of explicit promises from the seller that the product is new and genuine, and can be returned for a full refund if the buyer is not fully satisfied. 3.(MAX) Subjects were asked the amount that they would be willing to pay for the product from each of the described sellers, in the presence of explicit promises from the seller that the product is new and genuine, and can be returned for a full refund if the buyer is not satisfied. In addition, sellers were stated to be bonded. Funds for refund are guaran-teed to be available, and refunds were administered by a trusted third party with access to an escrow account, instead of by the seller. Those subjects who were exposed to treatments MIN and MID were considered to have been exposed to the MIN-MID test. Likewise, subjects who were exposed to MIN and MAX were referred to as MIN-MAX and those who were exposed to MID and MAX were referred to as MID-MAX. Through Qualtrics, subjects were randomly assigned to one of the tests. All of the subjects came from behavioral labs at each of the Universities associated with the experiment: the Wharton Behavioral lab, the Singapore Management University Lab, the Ludwig Maximilian University Lab, and Peking University Guanghua School of Man-agement Lab. The subjects recruited for this experi-ment were students at the participating schools. In order to determine the different types of ven-dors that exist in the e-commerce ecosystem, we did extensive online research to determine where certain products could be purchased. Using models from previous academic publications, we developed six vendor descriptions designed so that subjects would perceive them with differing levels of riskiness. The sellers were described as shown below. The phrases in parentheses were used as short identifiers of the ven-dors throughout the experiment. 1.(Safe Vendor) A well-known online seller in the country in which the experiment is being per-formed, with a strong reputation, such as Amazon, or a website associated with a well-known physical seller, such as Wal-Mart or Carrefour.2.(Favorite Vendor) A website in the country in which the experiment is being performed, that is a favorite of the subject. 3.(Friend’s Favorite Vendor) A website in the country in which the experiment is being per-formed, that the subject has not used before, but is strongly recommended by close personal friends. 4.(Unknown Rated Vendor) A website in the coun-try in which the experiment is being performed, that neither the subject nor friends have used be-fore, but that has a strong online rating. 5.(Unknown Unrated Vendor) A website in the country in which the experiment is being per-formed, that neither the subject nor friends have used before, and that does not have an online rat-ing. We will post the full vendor descriptions included in the experiment and a screenshot of an experiment page online, as they appeared to the subjects. Product selection involved analysis to ensure that products across test sites were of similar interest to subjects in different markets. Thus, in the sporting goods category, American and German students were offered an opportunity to buy tennis rackets, and in China and Singapore they were offered a table tennis paddle or running shoes. Categories included sporting equipment, high and low end consumer electronics, cosmetics, clothing, and food. We presented the subjects with two prices for each product, Manufacturers suggested retail price (MSRP) and Average Online Retail Price. We defined MSRP as the price that the manufacturers recommended for their products, and this price could often be found at the manufacturer’s own website or a trusted online seller’s website. We defined average online retail price as the price at which a trusted online seller like amazon.com or walmart.com lists the product for sale. Participants were informed that MSRP in the experiment is a proxy for the price a consumer would get at a physical full service store, which in some cases was similar to the price from the most trusted online vendor.
https://codeshoppy.com/shop/product/ediagnostic-lab-online-reporting-mobile-app/
Comments
Post a Comment